Pandas Series Series.value_counts() Function
-
Syntax of
pandas.Series.value_counts()
: -
Example Codes: Count Occurences of Unique Elements in Pandas Series Using
Series.value_counts()
Method -
Example Codes: Set
normalize=True
inSeries.value_counts()
Method to Obtain Relative Frequencies of Elements -
Example Codes: Set
ascending=True
inSeries.value_counts()
Method to Sort Elements Based on Frequency Value in Ascending Order -
Example Codes: Set
bins
Parameter inSeries.value_counts()
Method to Obtain Count of Values Lying in Half-Open Bins -
Example Codes: Set
dropna=False
inSeries.value_counts()
Method to CountsNaN
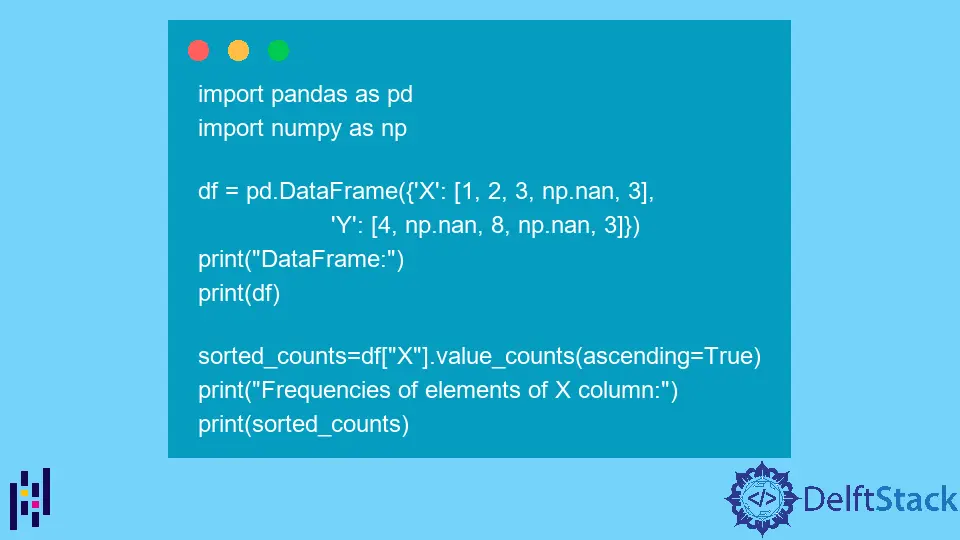
pandas.Series.value_counts()
method counts the number of occurrences of each unique element in the Series
.
Syntax of pandas.Series.value_counts()
:
Series.value_counts(normalize=False, sort=True, ascending=False, bins=None, dropna=True)
Parameters
normalize |
Boolean. Relative frequencies of the unique values(normalize=True ) or absolute frequencies of the unique values(normalize=False ). |
sort |
Boolean. Sort the elements based on frequencies(sort=True ) or leave the Series object unsorted(sort=False ) |
ascending |
Boolean. Sort the values in ascending order(ascending=True ) or descending order(ascending=False ) |
bins |
Integer. Number of partitions the range of values of Series object is divided into |
dropna |
Boolean. Include counts of NaN (dropna=False ) or exclude counts of NaN (dropna=True ) |
Return
It returns a Series object composed of the count of unique values.
Example Codes: Count Occurences of Unique Elements in Pandas Series Using Series.value_counts()
Method
import pandas as pd
import numpy as np
df = pd.DataFrame({'X': [1, 2, 3, np.nan, 3],
'Y': [4, np.nan, 8, np.nan, 3]})
print("DataFrame:")
print(df)
absolute_counts=df["X"].value_counts()
print("Frequencies of elements of X column:")
print(absolute_counts)
Output:
DataFrame:
X Y
0 1.0 4.0
1 2.0 NaN
2 3.0 8.0
3 NaN NaN
4 3.0 3.0
Frequencies of elements of X column:
3.0 2
2.0 1
1.0 1
Name: X, dtype: int64
The absolute_counts
gives the count of each unique element of column X
using Series.value_counts()
method.
Series.value_counts()
doesn’t count NaN
by default. We will introduce how to count it in the following sections.
Example Codes: Set normalize=True
in Series.value_counts()
Method to Obtain Relative Frequencies of Elements
If we set normalize=True
in Series.value_counts()
method, we get relative frequencies of all the unique elements in Series
object.
import pandas as pd
import numpy as np
df = pd.DataFrame({'X': [1, 2, 3, np.nan, 3],
'Y': [4, np.nan, 8, np.nan, 3]})
print("DataFrame:")
print(df)
relative_counts=df["X"].value_counts(normalize=True)
print("Relative Frequencies of elements of X column:")
print(relative_counts)
Output:
DataFrame:
X Y
0 1.0 4.0
1 2.0 NaN
2 3.0 8.0
3 NaN NaN
4 3.0 3.0
Frequencies of elements of X column:
3.0 0.50
2.0 0.25
1.0 0.25
Name: X, dtype: float64
The relative_counts
Series object gives the relative frequencies of each unique element of column X
.
Relative frequencies are obtained by dividing all the absolute frequencies by the sum of absolute frequencies.
Example Codes: Set ascending=True
in Series.value_counts()
Method to Sort Elements Based on Frequency Value in Ascending Order
If we set ascending=True
in Series.value_counts()
method, we get Series
object with its elements sorted based on frequency values in ascending order.
By default, the values in Series object returned from Series.value_counts()
method are sorted in descending order based on frequency values.
import pandas as pd
import numpy as np
df = pd.DataFrame({'X': [1, 2, 3, np.nan, 3],
'Y': [4, np.nan, 8, np.nan, 3]})
print("DataFrame:")
print(df)
sorted_counts=df["X"].value_counts(ascending=True)
print("Frequencies of elements of X column:")
print(sorted_counts)
Output:
DataFrame:
X Y
0 1.0 4.0
1 2.0 NaN
2 3.0 8.0
3 NaN NaN
4 3.0 3.0
Frequencies of elements of X column:
1.0 1
2.0 1
3.0 2
Name: X, dtype: int64
It gives counts of each unique object in X
column with frequency values sorted in ascending order.
Example Codes: Set bins
Parameter in Series.value_counts()
Method to Obtain Count of Values Lying in Half-Open Bins
import pandas as pd
import numpy as np
df = pd.DataFrame({'X': [1, 2, 3, np.nan, 3, 4, 5],
'Y': [4, np.nan, 8, np.nan, 3, 2, 1]})
print("DataFrame:")
print(df)
counts=df["X"].value_counts(bins=3)
print("Frequencies:")
print(counts)
Output:
DataFrame:
X Y
0 1.0 4.0
1 2.0 NaN
2 3.0 8.0
3 NaN NaN
4 3.0 3.0
5 4.0 2.0
6 5.0 1.0
Frequencies:
(3.667, 5.0] 2
(2.333, 3.667] 2
(0.995, 2.333] 2
Name: X, dtype: int64
It divides the range of values in the Series
, i.e., column X
into three parts and returns counts of values lying in each half-opened bins.
Example Codes: Set dropna=False
in Series.value_counts()
Method to Counts NaN
If we set dropna=False
in Series.value_counts()
method, we also get counts of NaN
values.
import pandas as pd
import numpy as np
df = pd.DataFrame({'X': [1, 2, 3, np.nan, 3],
'Y': [4, np.nan, 8, np.nan, 3]})
print("DataFrame:")
print(df)
counts=df["Y"].value_counts(dropna=False)
print("Frequencies:")
print(counts)
Output:
DataFrame:
X Y
0 1.0 4.0
1 2.0 NaN
2 3.0 8.0
3 NaN NaN
4 3.0 3.0
Frequencies:
NaN 2
3.0 1
8.0 1
4.0 1
Name: Y, dtype: int64
It gives the count of each element in the Y
column of DataFrame
with the count of NaN
values.
Suraj Joshi is a backend software engineer at Matrice.ai.
LinkedIn