将函数应用于 Pandas DataFrame 中的多个列
Manav Narula
2020年12月19日
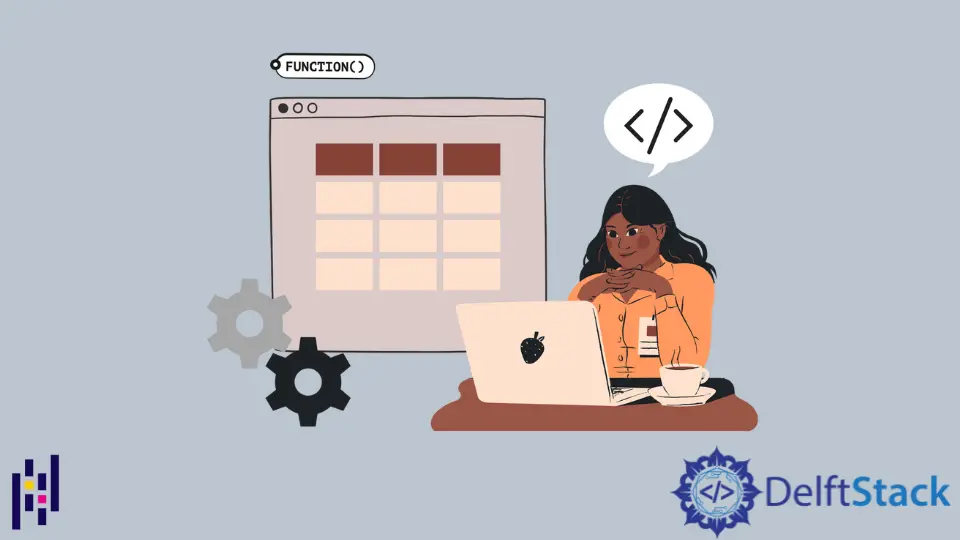
本文将介绍如何将函数应用于 Pandas DataFrame 中的多个列。在所有示例代码中,我们将使用以下相同的 DataFrame。
import pandas as pd
import numpy as np
df = pd.DataFrame(
[[5, 6, 7, 8], [1, 9, 12, 14], [4, 8, 10, 6]], columns=["a", "b", "c", "d"]
)
输出:
a b c d
0 5 6 7 8
1 1 9 12 14
2 4 8 10 6
使用 apply()
将函数应用到 Pandas 中的列
apply()
方法允许对整个 DataFrame 应用一个函数,可以跨列或跨行。我们将参数 axis
设置为 0 代表行,1 代表列。
在下面的例子中,我们将使用前面定义的函数来递增示例 DataFrame 的值。
import pandas as pd
import numpy as np
df = pd.DataFrame(
[[5, 6, 7, 8], [1, 9, 12, 14], [4, 8, 10, 6]], columns=["a", "b", "c", "d"]
)
def x(a):
return a + 1
df_new = df.apply(x, axis=1)
print("The original dataframe:")
print(df)
print("The new dataframe:")
print(df_new)
输出:
The original dataframe:
a b c d
0 5 6 7 8
1 1 9 12 14
2 4 8 10 6
The new dataframe:
a b c d
0 6 7 8 9
1 2 10 13 15
2 5 9 11 7
我们也可以将一个函数应用于多列,如下图所示。
import pandas as pd
import numpy as np
df = pd.DataFrame(
[[5, 6, 7, 8], [1, 9, 12, 14], [4, 8, 10, 6]], columns=["a", "b", "c", "d"]
)
print("The original dataframe:")
print(df)
def func(x):
return x[0] + x[1]
df["e"] = df.apply(func, axis=1)
print("The new dataframe:")
print(df)
输出:
The original dataframe:
a b c d
0 5 6 7 8
1 1 9 12 14
2 4 8 10 6
The new dataframe:
a b c d e
0 5 6 7 8 11
1 1 9 12 14 10
2 4 8 10 6 12
新添加的 e
列是 a
和 b
列中数据的总和。DataFrame 本身是传递给函数的隐藏参数。可以像上面的例子一样用索引来访问列,或者用列名来访问,如下所示。
import pandas as pd
import numpy as np
df = pd.DataFrame(
[[5, 6, 7, 8], [1, 9, 12, 14], [4, 8, 10, 6]], columns=["a", "b", "c", "d"]
)
print("The original dataframe:")
print(df)
df["e"] = df.apply(lambda x: x.a + x.b, axis=1)
print("The new dataframe:")
print(df)
它执行的操作和上面的例子一样。我们在这里使用了一个 lambda
函数。x.a
和 x.b
指的是 DataFrame 中的列 a
和 b
。
作者: Manav Narula
Manav is a IT Professional who has a lot of experience as a core developer in many live projects. He is an avid learner who enjoys learning new things and sharing his findings whenever possible.
LinkedIn