Python의 자동 ARIMA
Zeeshan Afridi
2023년10월10일
Python
Python ARIMA
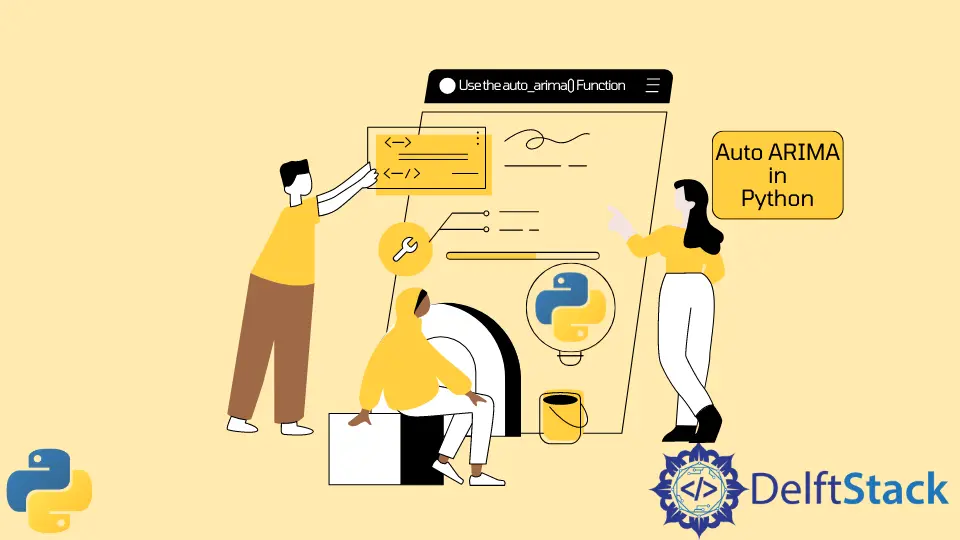
이 기사에서는 Python의 Auto ARIMA와 작동 방식에 대해 알아봅니다.
Python의 자동 ARIMA
pmdarima
라이브러리의 auto_arima()
함수는 ARIMA 모델의 최적 매개변수를 결정하는 데 도움을 주며 결과적으로 적합한 ARIMA 모델을 제공합니다.
이 패키지는 "pmdarima"
로 이름이 바뀌기 전에 "Pyramid"
라고 불렸습니다. "pmdarima"
패키지가 설치되고 있는지 확인하십시오.
패키지가 없는 경우 터미널에서 아래 명령을 실행하여 설치합니다.
pip install pmdarima
다음 명령을 사용하여 패키지가 성공적으로 생성되었는지 테스트합니다.
from pmdarima.arima import auto_arima
Python에서 auto_arima()
함수 사용
다음 코드에서 data.csv는 데이터가 포함된 CSV 파일이며 자동 ARIMA에 사용됩니다. 출력은 인덱스 p
및 q
에서 order=(P,D,Q)
값이 있는 데이터 프레임입니다.
코드 예:
import pmdarima as pm
import pandas as pd
df1 = pd.read_csv("data.csv", names=["value"], header=0)
model_1 = pm.auto_arima(
df1.value,
start_p=1,
start_q=1,
test="adf",
max_p=3,
max_q=3,
m=1,
d=None,
seasonal=False,
start_P=0,
D=0,
trace=True,
error_action="ignore",
suppress_warnings=True,
stepwise=True,
)
print(model_1.summary())
출력:
Performing stepwise search to minimize aic
ARIMA(1,1,1)(0,0,0)[0] intercept : AIC=1605.366, Time=0.09 sec
ARIMA(0,1,0)(0,0,0)[0] intercept : AIC=1660.860, Time=0.01 sec
ARIMA(1,1,0)(0,0,0)[0] intercept : AIC=1619.269, Time=0.04 sec
ARIMA(0,1,1)(0,0,0)[0] intercept : AIC=1604.209, Time=0.04 sec
ARIMA(0,1,0)(0,0,0)[0] : AIC=1658.968, Time=0.01 sec
ARIMA(0,1,2)(0,0,0)[0] intercept : AIC=1605.215, Time=0.08 sec
ARIMA(1,1,2)(0,0,0)[0] intercept : AIC=1606.845, Time=0.12 sec
ARIMA(0,1,1)(0,0,0)[0] : AIC=1603.295, Time=0.02 sec
ARIMA(1,1,1)(0,0,0)[0] : AIC=1604.373, Time=0.03 sec
ARIMA(0,1,2)(0,0,0)[0] : AIC=1604.196, Time=0.04 sec
ARIMA(1,1,0)(0,0,0)[0] : AIC=1617.588, Time=0.04 sec
ARIMA(1,1,2)(0,0,0)[0] : AIC=1605.883, Time=0.04 sec
Best model: ARIMA(0,1,1)(0,0,0)[0]
Total fit time: 0.580 seconds
SARIMAX Results
==============================================================================
Dep. Variable: y No. Observations: 173
Model: SARIMAX(0, 1, 1) Log Likelihood -799.648
Date: Sat, 03 Sep 2022 AIC 1603.295
Time: 23:15:18 BIC 1609.590
Sample: 0 HQIC 1605.849
- 173
Covariance Type: opg
==============================================================================
coef std err z P>|z| [0.025 0.975]
------------------------------------------------------------------------------
ma.L1 -0.5856 0.056 -10.478 0.000 -0.695 -0.476
sigma2 637.6579 54.893 11.616 0.000 530.069 745.247
===================================================================================
Ljung-Box (L1) (Q): 0.54 Jarque-Bera (JB): 24.81
Prob(Q): 0.46 Prob(JB): 0.00
Heteroskedasticity (H): 0.18 Skew: 0.41
Prob(H) (two-sided): 0.00 Kurtosis: 4.67
===================================================================================
Process finished with exit code 0
결론
ARIMA 모델은 향후 며칠 동안 주식 실적을 광범위하게 추정합니다. Python의 auto_arima()
함수는 피팅된 ARIMA 모델의 최적 매개변수를 식별하는 데 사용됩니다.
auto_arima()
함수는 pmdarima
라는 Python 라이브러리에서 가져올 수 있습니다.
튜토리얼이 마음에 드시나요? DelftStack을 구독하세요 YouTube에서 저희가 더 많은 고품질 비디오 가이드를 제작할 수 있도록 지원해주세요. 구독하다
작가: Zeeshan Afridi
Zeeshan is a detail oriented software engineer that helps companies and individuals make their lives and easier with software solutions.
LinkedIn