How to Perform Logistic Regression in Python
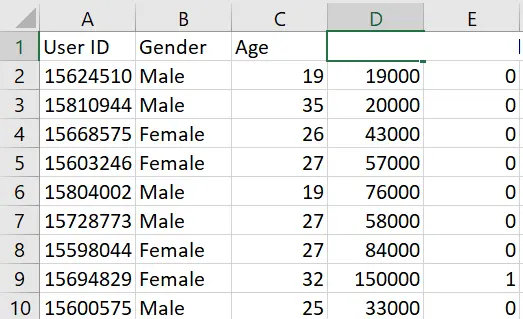
The following tutorial demonstrates how to perform logistic regression on Python.
Let us download a sample dataset to get started with. We will use a user dataset containing information about the user’s gender, age, and salary and predict if a user will eventually buy the product.
Take a look at our dataset.
We will now start creating our model by importing pertinent libraries such as pandas
, numpy
and matplotlib
.
Perform Logistic Regression in Python
Importing pertinent libraries:
import pandas as pd
import numpy as np
import matplotlib.pyplot as plt
Let us import our dataset using pandas
.
Reading dataset:
dataset = pd.read_csv("log_data.csv")
We will now select the Age
and Estimated salary
features from our dataset to train our model to predict if a user purchases a product or not. Here, gender
and user id
won’t play a significant role in predicting; we ignore them in the training process.
x = dataset.iloc[:, [2, 3]].values
y = dataset.iloc[:, 4].values
Let’s split the dataset into training and testing data. We divide them into 75% for training the model and the rest 25% for testing the model’s performance.
We do this using train_test_split
function in sklearn
library.
from sklearn.model_selection import train_test_split
xtrain, xtest, ytrain, ytest = train_test_split(x, y, test_size=0.25, random_state=0)
We perform the feature scaling process since the Age
and Salary
features lie in a different range. This is essential since one feature can dominate the other while the training process is avoided.
from sklearn.preprocessing import StandardScaler
sc_x = StandardScaler()
xtrain = sc_x.fit_transform(xtrain)
xtest = sc_x.transform(xtest)
Both the features lie in the range from -1 to 1, which will ensure both features contribute equally to decision-making (i.e., predicting process). Let us take a look at updated features.
print(xtrain[0:10, :])
[[ 0.58164944 -0.88670699]
[-0.60673761 1.46173768]
[-0.01254409 -0.5677824 ]
[-0.60673761 1.89663484]
[ 1.37390747 -1.40858358]
[ 1.47293972 0.99784738]
[ 0.08648817 -0.79972756]
[-0.01254409 -0.24885782]
[-0.21060859 -0.5677824 ]
[-0.21060859 -0.19087153]]
Let us finally train our model; in our case, we will use the logistic regression model, which we will import from the sklearn
library.
from sklearn.linear_model import LogisticRegression
classifier1 = LogisticRegression(random_state=0)
classifier1.fit(xtrain, ytrain)
Since we have now trained our model, let us do the prediction on our testing data to evaluate our model.
y_pred = classifier1.predict(xtest)
Let us now create a confusion matrix based on our testing data and the predictions we obtained in the last procedure.
from sklearn.metrics import confusion_matrix
cm = confusion_matrix(ytest, y_pred)
print("Confusion Matrix : \n", cm)
Confusion Matrix :
[[65 3]
[ 8 24]]
Let us calculate the accuracy of our model using the sklearn
library.
from sklearn.metrics import accuracy_score
print("Accuracy score : ", accuracy_score(ytest, y_pred))
Accuracy score : 0.89
We got a satisfying accuracy score of 0.89
from our model, which signifies that our model can very well predict if a user will buy a product or not.
Thus, we can successfully perform logistic regression using Python with the above method.