How to Find Duplicate Rows in a DataFrame Using Pandas
-
Use the
DataFrame.duplicated()
Method to Find Duplicate Rows in a DataFrame - Create a DataFrame With Duplicate Rows
- Select Duplicate Rows Based on All Columns
- Conclusion
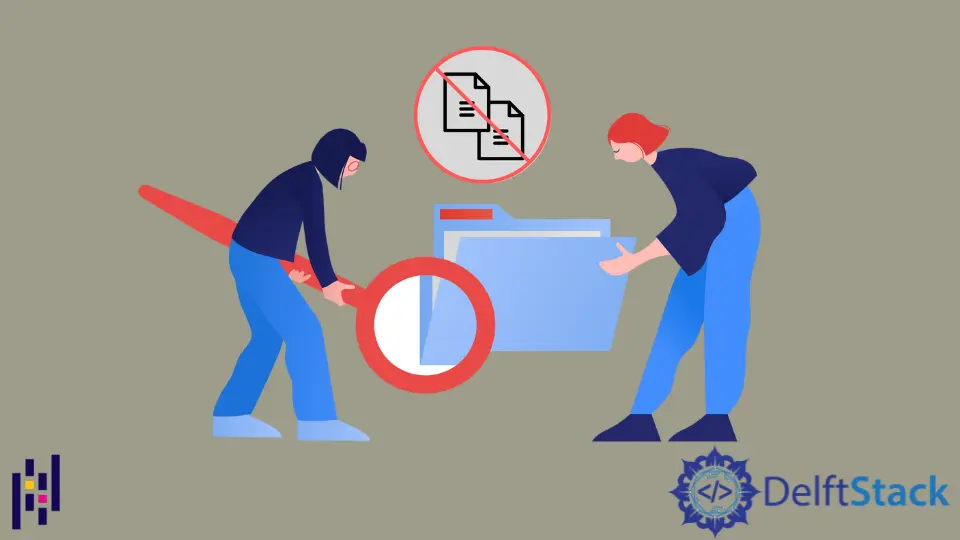
Duplicate values should be identified from your data set as part of the cleaning procedure. Duplicate data consumes unnecessary storage space and, at the very least, slows down calculations; however, in the worst-case scenario, duplicate data can distort analysis results and compromise the data set’s integrity.
An open-source Python package called Pandas enhances the handling and storage of structured data. Additionally, the framework offers built-in assistance for data cleaning procedures, such as finding and deleting duplicate rows and columns.
This article describes finding duplicates in a Pandas dataframe using all or a subset of the columns. For this, we will use the Dataframe.duplicated()
method of Pandas.
Use the DataFrame.duplicated()
Method to Find Duplicate Rows in a DataFrame
The Pandas library for Python’s DataFrame
class offers a member method to discover duplicate rows based on either all columns or a subset of those columns, such as:
DataFrame.duplicated(subset=None, keep="first")
It gives back a series of booleans indicating whether a row is duplicate or unique.
Parameters:
subset
: This requires a column or collection of column labels. None is the default value for it. After passing columns, it will only take duplicates into account.keep
: This regulates the treatment of duplicate values. There are only three different values, withfirst
being the default.- If
first
, the first item is treated as unique and the remaining values as duplicates. - If
latest
, the final item is treated as unique and the remaining values as duplicates. - If
False
, all identical values are regarded as duplicates. - It returns the duplicate rows indicated by the boolean series.
Create a DataFrame With Duplicate Rows
Let’s make a basic Dataframe with a collection of lists and name the columns Name
, Age
, and City
.
Example Code:
# Import pandas library
import pandas as pd
# List of Tuples
employees = [
("Joe", 28, "Chicago"),
("John", 32, "Austin"),
("Melvin", 25, "Dallas"),
("John", 32, "Austin"),
("John", 32, "Austin"),
("John", 32, "Houston"),
("Melvin", 40, "Dehradun"),
("Hazel", 32, "Austin"),
]
df = pd.DataFrame(employees, columns=["Name", "Age", "City"])
print(df)
Output:
Name Age City
0 Joe 28 Chicago
1 John 32 Austin
2 Melvin 25 Dallas
3 John 32 Austin
4 John 32 Austin
5 John 32 Houston
6 Melvin 40 Dehradun
7 Hazel 32 Austin
Select Duplicate Rows Based on All Columns
Call Dataframe.duplicate()
without a subset
parameter to locate and select duplicates for all rows depending on all columns. However, if there are duplicate rows, it will only return a Boolean series with True
at the first instance’s location (the default value of the retain argument is first
).
Then give this Boolean Series to the DataFrame’s []
operator to choose the duplicate rows.
Example Code:
# Import pandas library
import pandas as pd
# List of Tuples
employees = [
("Joe", 28, "Chicago"),
("John", 32, "Austin"),
("Melvin", 25, "Dallas"),
("John", 32, "Austin"),
("John", 32, "Austin"),
("John", 32, "Houston"),
("Melvin", 40, "Dehradun"),
("Hazel", 32, "Austin"),
]
df = pd.DataFrame(employees, columns=["Name", "Age", "City"])
duplicate = df[df.duplicated()]
print("Duplicate Rows :")
print(duplicate)
Output:
Duplicate Rows :
Name Age City
3 John 32 Austin
4 John 32 Austin
Pass retain = "last"
as an argument if you want to consider all duplicates except the final one.
Example Code:
# Import pandas library
import pandas as pd
# List of Tuples
employees = [
("Joe", 28, "Chicago"),
("John", 32, "Austin"),
("Melvin", 25, "Dallas"),
("John", 32, "Austin"),
("John", 32, "Austin"),
("John", 32, "Houston"),
("Melvin", 40, "Dehradun"),
("Hazel", 32, "Austin"),
]
df = pd.DataFrame(employees, columns=["Name", "Age", "City"])
duplicate = df[df.duplicated(keep="last")]
print("Duplicate Rows :")
print(duplicate)
Output:
Duplicate Rows :
Name Age City
1 John 32 Austin
3 John 32 Austin
Then, provide the list of column names in the subset
as a parameter if you only want to select duplicate rows depending on a few specified columns.
Example Code:
# Import pandas library
import pandas as pd
# List of Tuples
employees = [
("Joe", 28, "Chicago"),
("John", 32, "Austin"),
("Melvin", 25, "Dallas"),
("John", 32, "Austin"),
("John", 32, "Austin"),
("John", 32, "Houston"),
("Melvin", 40, "Dehradun"),
("Hazel", 32, "Austin"),
]
df = pd.DataFrame(employees, columns=["Name", "Age", "City"])
# on 'City' column
duplicate = df[df.duplicated("City")]
print("Duplicate Rows based on City:")
print(duplicate)
Output:
Duplicate Rows based on City:
Name Age City
3 John 32 Austin
4 John 32 Austin
7 Hazel 32 Austin
Select the duplicate rows based on more than one column name, such as Name
and Age
.
Example Code:
# Import pandas library
import pandas as pd
# List of Tuples
employees = [
("Joe", 28, "Chicago"),
("John", 32, "Austin"),
("Melvin", 25, "Dallas"),
("John", 32, "Austin"),
("John", 32, "Austin"),
("John", 32, "Houston"),
("Melvin", 40, "Dehradun"),
("Hazel", 32, "Austin"),
]
df = pd.DataFrame(employees, columns=["Name", "Age", "City"])
# list of the column names
duplicate = df[df.duplicated(["Name", "Age"])]
print("Duplicate the rows based on Name and Age:")
print(duplicate)
Output:
Duplicate Rows based on Name and Age:
Name Age City
3 John 32 Austin
4 John 32 Austin
5 John 32 Houston
Conclusion
To locate duplicate rows in a DataFrame, use the dataframe.duplicated()
method in Pandas. It gives back a series of booleans indicating whether a row is duplicate or unique.
We hope this article has helped you find duplicate rows in a Dataframe using all or a subset of the columns by checking all the examples we have discussed here. Then, using the above-discussed easy steps, you can quickly determine how Pandas can be used to find duplicates.
Zeeshan is a detail oriented software engineer that helps companies and individuals make their lives and easier with software solutions.
LinkedIn