Pandas Axis Meaning
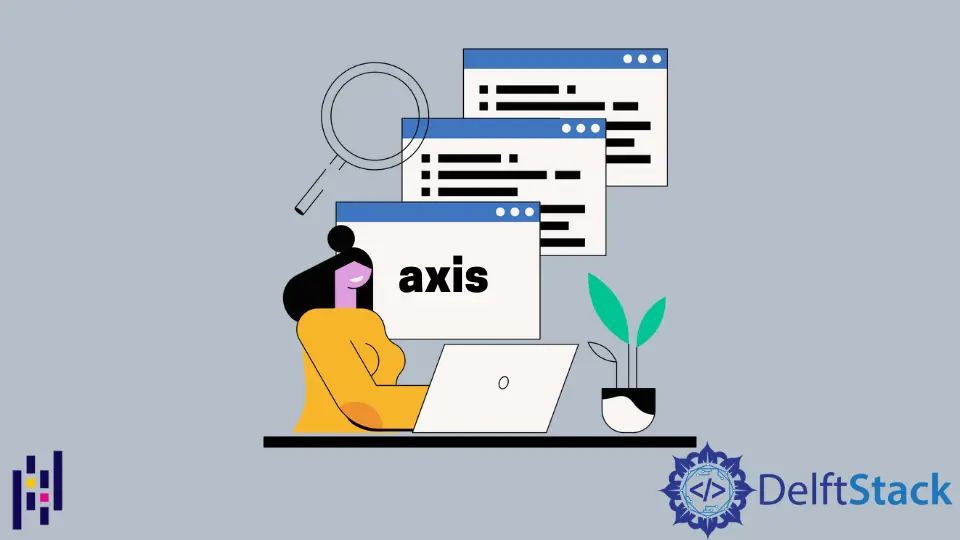
This tutorial explains the meaning of the axis
parameter used in various methods of Pandas objects like DataFrames and Series
.
import pandas as pd
empl_df = pd.DataFrame(
{
"Name": ["Jon", "Willy", "Mike", "Luna", "Sam", "Aliza"],
"Age": [30, 33, 35, 30, 30, 31],
"Weight(KG)": [75, 75, 80, 70, 73, 70],
"Height(meters)": [1.7, 1.7, 1.85, 1.75, 1.8, 1.75],
"Salary($)": [3300, 3500, 4000, 3050, 3500, 3700],
}
)
print(empl_df)
Output:
Name Age Weight(KG) Height(meters) Salary($)
0 Jon 30 75 1.70 3300
1 Willy 33 75 1.70 3500
2 Mike 35 80 1.85 4000
3 Luna 30 70 1.75 3050
4 Sam 30 73 1.80 3500
5 Aliza 31 70 1.75 3700
We use the DataFrame empl_df
to explain how to use the axis
parameter in Pandas methods.
Use of axis
Parameter in Pandas Methods
The axis
parameter specifies the direction along which a particular method or function is applied in a DataFrame. axis=0
represents the function is applied column-wise, and axis=1
means that the function is applied row-wise on the DataFrame.
If we apply a function column-wise, we will get a result with a single row; if we apply a function row-wise, we will get a DataFrame with a single column.
Example: Use axis=0
in Pandas Methods
import pandas as pd
empl_df = pd.DataFrame(
{
"Name": ["Jon", "Willy", "Mike", "Luna", "Sam", "Aliza"],
"Age": [30, 33, 35, 30, 30, 31],
"Weight(KG)": [75, 75, 80, 70, 73, 70],
"Height(meters)": [1.7, 1.7, 1.85, 1.75, 1.8, 1.75],
"Salary($)": [3300, 3500, 4000, 3050, 3500, 3700],
}
)
print("The Employee DataFrame is:")
print(empl_df, "\n")
print("The DataFrame with mean values of each column is:")
print(empl_df.mean(axis=0))
Output:
The Employee DataFrame is:
Name Age Weight(KG) Height(meters) Salary($)
0 Jon 30 75 1.70 3300
1 Willy 33 75 1.70 3500
2 Mike 35 80 1.85 4000
3 Luna 30 70 1.75 3050
4 Sam 30 73 1.80 3500
5 Aliza 31 70 1.75 3700
The DataFrame with mean values of each column is:
Age 31.500000
Weight(KG) 73.833333
Height(meters) 1.758333
Salary($) 3508.333333
dtype: float64
It calculates the column-wise mean of the DataFrame empl_df
. The mean is calculated only for columns with numerical values.
If we set axis=0
, it will calculate each column’s mean by averaging the row values for that particular column.
Example: Use axis=1
in Pandas Methods
import pandas as pd
empl_df = pd.DataFrame(
{
"Name": ["Jon", "Willy", "Mike", "Luna", "Sam", "Aliza"],
"Age": [30, 33, 35, 30, 30, 31],
"Weight(KG)": [75, 75, 80, 70, 73, 70],
"Height(meters)": [1.7, 1.7, 1.85, 1.75, 1.8, 1.75],
"Salary($)": [3300, 3500, 4000, 3050, 3500, 3700],
}
)
print("The Employee DataFrame is:")
print(empl_df, "\n")
print("The DataFrame with mean values of each row is:")
print(empl_df.mean(axis=1))
Output:
The Employee DataFrame is:
Name Age Weight(KG) Height(meters) Salary($)
0 Jon 30 75 1.70 3300
1 Willy 33 75 1.70 3500
2 Mike 35 80 1.85 4000
3 Luna 30 70 1.75 3050
4 Sam 30 73 1.80 3500
5 Aliza 31 70 1.75 3700
The DataFrame with mean values of each row is:
0 851.6750
1 902.4250
2 1029.2125
3 787.9375
4 901.2000
5 950.6875
dtype: float64
It calculates row-wise mean for the DataFrame empl_df
, in other words, it will calculate the mean value for each row by averaging the column values of numeric type for that row. We will get a single column at the end with the average value for each row.
Suraj Joshi is a backend software engineer at Matrice.ai.
LinkedInRelated Article - Pandas DataFrame Row
- How to Get the Row Count of a Pandas DataFrame
- How to Randomly Shuffle DataFrame Rows in Pandas
- How to Filter Dataframe Rows Based on Column Values in Pandas
- How to Iterate Through Rows of a DataFrame in Pandas
- How to Get Index of All Rows Whose Particular Column Satisfies Given Condition in Pandas
- How to Find Duplicate Rows in a DataFrame Using Pandas