How to Get the Aggregate of Pandas Group-By and Sum
-
Cumulative Sum With
groupby
-
pivot()
to Rearrange the Data in a Nice Table -
Apply
function
togroupby
in Pandas -
agg()
to Get Aggregate Sum of the Column
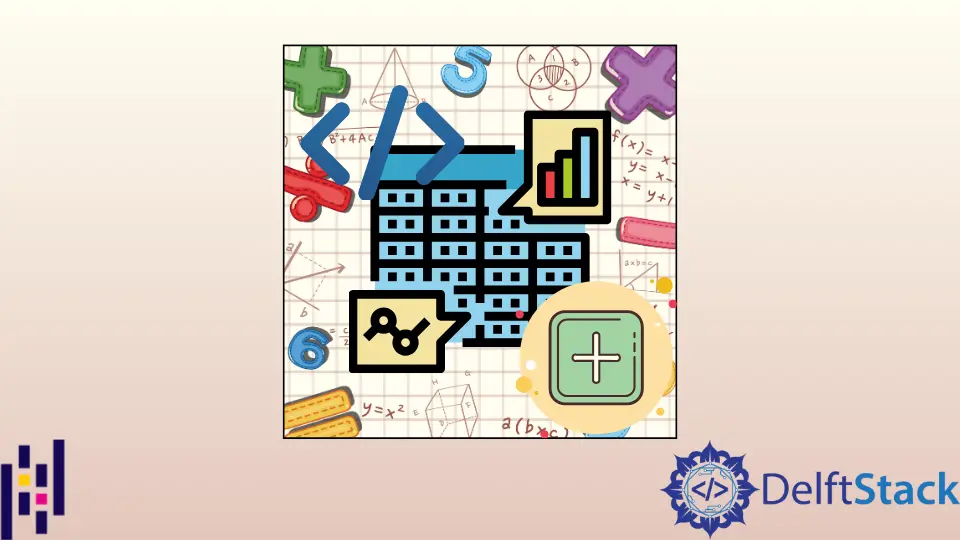
We will demonstrate how to get the aggregate in Pandas by using groupby
and sum
. We will also look at the pivot
functionality to arrange the data in a nice table and define our custom function and run it on the DataFrame
. We will also get the aggregate sum by using agg()
.
Cumulative Sum With groupby
We can get the cumulative sum by using groupby
method. Consider the following Dataframe with Date
, Fruit
and Sale
columns:
import pandas as pd
df = pd.DataFrame(
{
"Date": ["08/09/2018", "10/09/2018", "08/09/2018", "10/09/2018"],
"Fruit": [
"Apple",
"Apple",
"Banana",
"Banana",
],
"Sale": [34, 12, 22, 27],
}
)
If we want to calculate the cumulative sum of Sale per Fruit and for every date, we can do:
import pandas as pd
df = pd.DataFrame(
{
"Date": ["08/09/2018", "10/09/2018", "08/09/2018", "10/09/2018"],
"Fruit": [
"Apple",
"Apple",
"Banana",
"Banana",
],
"Sale": [34, 12, 22, 27],
}
)
print(df.groupby(by=["Fruit", "Date"]).sum().groupby(level=[0]).cumsum())
Output:
Fruit Date Sale
Apple 08/09/2018 34
10/09/2018 46
Banana 08/09/2018 22
10/09/2018 49
pivot()
to Rearrange the Data in a Nice Table
pivot()
method could set row and column attribute of the table. Let’s change the above code and apply pivot()
method to rearrange the data in a nice table:
import pandas as pd
df = pd.DataFrame(
{
"Date": ["08/09/2018", "10/09/2018", "08/09/2018", "10/09/2018"],
"Fruit": [
"Apple",
"Apple",
"Banana",
"Banana",
],
"Sale": [34, 12, 22, 27],
}
)
print(
df.groupby(["Fruit", "Date"], as_index=False).sum().pivot("Fruit", "Date").fillna(0)
)
Output:
Sale
Date 08/09/2018 10/09/2018
Fruit
Apple 34 12
Banana 22 27
Apply function
to groupby
in Pandas
We will create a simple method to get count of values in series
or 1d array
and use groupby
to get aggregate count of each value:
from pandas import *
d = {"series": Series(["1", "2", "1", "1", "4", "4", "5"])}
df = DataFrame(d)
def get_count(values):
return len(values)
grouped_count = df.groupby("series").series.agg(get_count)
print(grouped_count)
After running the code, we will get the following output, which provides each value’s occurrence in the series.
Output:
series
1 3
2 1
4 2
5 1
Name: series, dtype: int64
agg()
to Get Aggregate Sum of the Column
We can use agg()
to apply sum operation on column. Example Codes:
import pandas as pd
df = pd.DataFrame(
{
"Date": ["08/09/2018", "10/09/2018", "08/09/2018", "10/09/2018"],
"Fruit": [
"Apple",
"Apple",
"Banana",
"Banana",
],
"Sale": [34, 12, 22, 27],
}
)
print(df.groupby(["Fruit"])["Sale"].agg("sum"))
Output:
Fruit
Apple 46
Banana 49
Name: Sale, dtype: int64