How to Apply a Function to a Column in Pandas Dataframe
-
Pandas
apply()
andtransform()
Methods -
Use
apply()
to Apply a Function to Pandas DataFrame Column -
Use
transform()
to Apply a Function to Pandas DataFrame Column
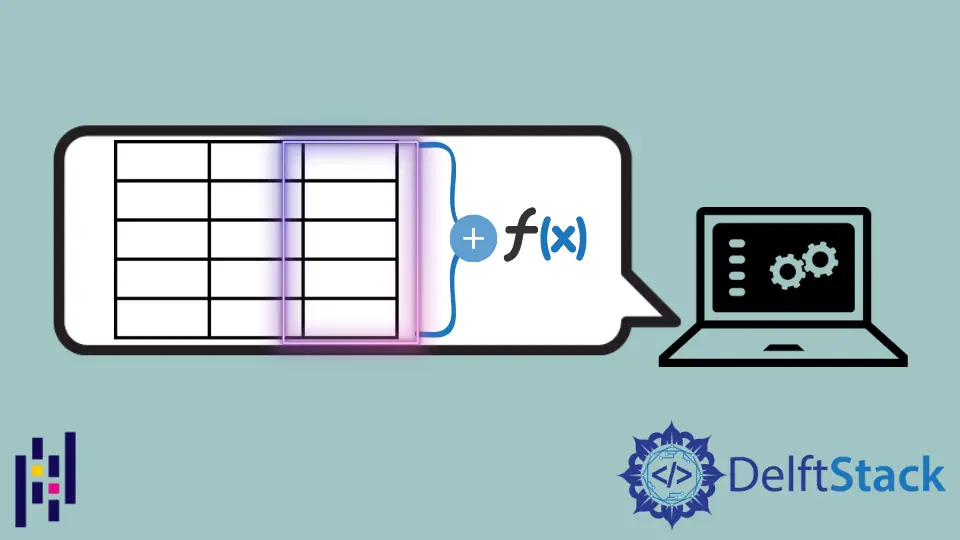
In Pandas, columns and dataframes can be transformed and manipulated using methods such as apply()
and transform()
. The desired transformations are passed in as arguments to the methods as functions. Each method has its subtle differences and utility.
This article will introduce how to apply a function to a column or an entire dataframe.
Pandas apply()
and transform()
Methods
Both apply()
and transform()
methods operate on individual columns and the whole dataframe. The apply()
method applies the function along a specified axis. It passes the columns as a dataframe to the custom function, whereas a transform()
method passes individual columns as pandas Series
to the custom function.
The apply()
method’s output is received in the form of a dataframe or Series
depending on the input, whereas as a sequence for the transform()
method. Both the syntax of the apply()
and transform()
methods resemble the same as:
Dataframe.apply(customFunction, axis=0)
Dataframe.transform(customFunction, axis=0)
The arguments correspond to
customFunction
: the function to be applied to the dataframe or series.axis
: 0 refers to'rows'
, and 1 refers to'columns'
; the function needs to be applied on either rows or columns.
Use apply()
to Apply a Function to Pandas DataFrame Column
Now we have mastered the basics, let’s get our hands on the codes and understand how to use the apply()
method to apply a function to a dataframe column.
We will use the example dataframe as below.
import pandas as pd
import numpy as np
df = pd.DataFrame([[1, 2, 3], [4, 5, 6], [7, 8, 9]], columns=["A", "B", "C"])
print(df)
The example code to apply a function to the whole DataFrame is shown below.
import pandas as pd
import numpy as np
df = pd.DataFrame([[1, 2, 3], [4, 5, 6], [7, 8, 9]], columns=["A", "B", "C"])
print(df)
def add_2(x):
return x + 2
df = df.apply(add_2)
print(df)
Output:
A B C
0 1 2 3
1 4 5 6
2 7 8 9
A B C
0 3 4 5
1 6 7 8
2 9 10 11
As seen above, the function can be applied for the whole of the dataframe.
Apply a Function to a Single Column
Let’s see what happens when the function is applied along a single column.
import pandas as pd
import numpy as np
df = pd.DataFrame([[1, 2, 3], [4, 5, 6], [7, 8, 9]], columns=["A", "B", "C"])
print(df)
def add_2(x):
return x + 2
df["A"] = df["A"].apply(add_2)
print(df)
# or #
df["A"].transform(add_2)
print(df)
Output:
A B C
0 1 2 3
1 4 5 6
2 7 8 9
A B C
0 3 2 3
1 6 5 6
2 9 8 9
Another Example of Applying a Function to a Single Column
import numpy as np
import pandas as pd
roll_no = [501, 502, 503, 504, 505]
data = pd.DataFrame(
{"A": [20, 30, 15, 25, 20], "B": [4, 5, 6, 4, 6], "C": [12, 15, 13, 12, 14]}
)
print("Initial DataFrame:")
print(data)
print("")
updated_df = data.apply(lambda x: x - 5 if x.name == "A" else x)
print("Updated DataFrame:")
print(updated_df)
Output:
Initial DataFrame:
A B C
0 20 4 12
1 30 5 15
2 15 6 13
3 25 4 12
4 20 6 14
Updated DataFrame:
A B C
0 15 4 12
1 25 5 15
2 10 6 13
3 20 4 12
4 15 6 14
Here, we apply the lambda
function defined for each column in the DataFrame. The function subtracts every column’s value by 5
only if the name of the column is A
.
import numpy as np
import pandas as pd
roll_no = [501, 502, 503, 504, 505]
data = pd.DataFrame(
{"A": [20, 30, 15, 25, 20], "B": [4, 5, 6, 4, 6], "C": [12, 15, 13, 12, 14]}
)
print("Initial DataFrame:")
print(data)
print("")
data["A"] = data["A"].apply(lambda x: x - 5)
print("Updated DataFrame:")
print(data)
Output:
Initial DataFrame:
A B C
0 20 4 12
1 30 5 15
2 15 6 13
3 25 4 12
4 20 6 14
Updated DataFrame:
A B C
0 15 4 12
1 25 5 15
2 10 6 13
3 20 4 12
4 15 6 14
It applies the lambda
function only to the column A
of the DataFrame, and we finally assign the returned values back to column A
of the existing DataFrame.
Use transform()
to Apply a Function to Pandas DataFrame Column
Let’s see how to use the transform()
method to apply a function to a dataframe column. We will use the same example dataframe as above.
The example code to apply a function to the whole DataFrame is shown below.
import pandas as pd
import numpy as np
df = pd.DataFrame([[1, 2, 3], [4, 5, 6], [7, 8, 9]], columns=["A", "B", "C"])
print(df)
def add_2(x):
return x + 2
df = df.transform(add_2)
print(df)
Output:
A B C
0 1 2 3
1 4 5 6
2 7 8 9
A B C
0 3 4 5
1 6 7 8
2 9 10 11
As seen above, the function can be applied to the whole dataframe.
Apply a Function to a Single Column
Let’s see what happens when the function is applied along a single column.
import pandas as pd
import numpy as np
df = pd.DataFrame([[1, 2, 3], [4, 5, 6], [7, 8, 9]], columns=["A", "B", "C"])
print(df)
def add_2(x):
return x + 2
df["A"] = df["A"].transform(add_2)
print(df)
Output:
A B C
0 1 2 3
1 4 5 6
2 7 8 9
A B C
0 3 2 3
1 6 5 6
2 9 8 9