Pandas pandas.melt() Function
-
Syntax of
pandas.melt()
-
Example Codes:
pandas.melt()
-
Example Codes:
pandas.melt()
With Single Column asid_vars
-
Example Codes:
pandas.melt()
With Skipping Columns -
Example Codes:
pandas.melt()
With Multiple Columns
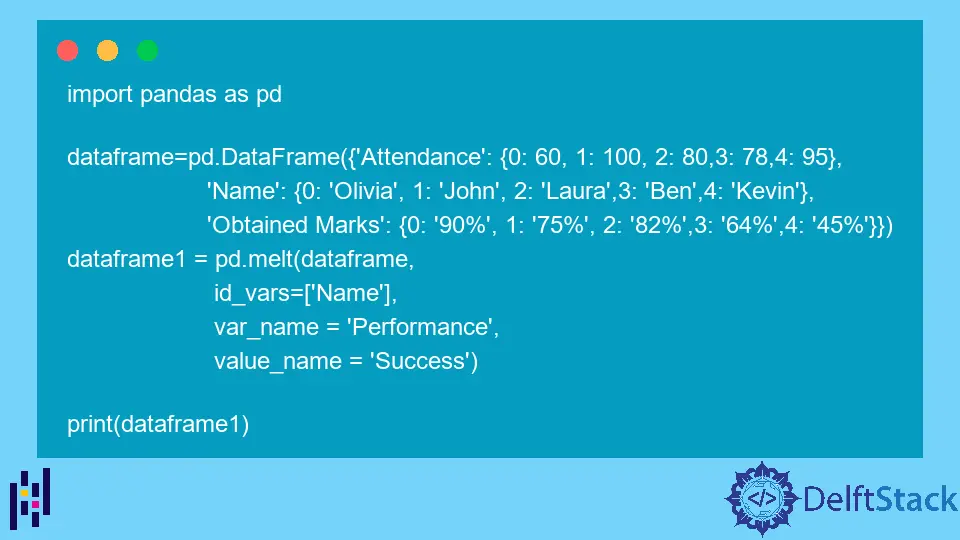
pandas.melt()
function reshapes or transforms an existing DataFrame
. It changes the orientation of the DataFrame
from a wide format to a long format.
Syntax of pandas.melt()
pandas.melt(dataframe, id_vars, value_vars, var_name, value_name, col_level)
Parameters
Dataframe |
mandatory | It is the DataFrame that we wish to change into the long format. |
id_vars |
optional | It can be a tuple , list , or an N-dimensional array. It is the column used for identifier variables. You can select more than one identifier column. |
value_vars |
optional | It can be a tuple , list , or an N-dimensional array. By default, the columns not specified as identifier variables are value variables. You can also select these. |
var_name |
optional | It is a scalar type variable. It is the name of the identifier column. By default, it is variable . |
value_name |
optional | It is a scalar type variable. It is the name of the non-identifier column. By default, it is value . |
col_level |
optional | It is an integer or a string. In the case of multi-index columns, we can use this parameter to transform our DataFrame . |
Return
It returns a transformed DataFrame
that contains one or more identifier columns and only two non-identifier columns named variable
and value
.
Example Codes: pandas.melt()
At first, we will check this function by only passing the mandatory parameter i.e DataFrame
.
import pandas as pd
dataframe=pd.DataFrame({'Attendance': {0: 60, 1: 100, 2: 80,3: 78,4: 95},
'Name': {0: 'Olivia', 1: 'John', 2: 'Laura',3: 'Ben',4: 'Kevin'},
'Obtained Marks': {0: '90%', 1: '75%', 2: '82%',3: '64%',4: '45%'}})
dataframe1 = pd.melt(dataframe)
print(dataframe1)
The example DataFrame
is as below.
Attendance Name Obtained Marks
0 60 Olivia 90%
1 100 John 75%
2 80 Laura 82%
3 78 Ben 64%
4 95 Kevin 45%
Output:
variable value
0 Attendance 60
1 Attendance 100
2 Attendance 80
3 Attendance 78
4 Attendance 95
5 Name Olivia
6 Name John
7 Name Laura
8 Name Ben
9 Name Kevin
10 Obtained Marks 90%
11 Obtained Marks 75%
12 Obtained Marks 82%
13 Obtained Marks 64%
14 Obtained Marks 45%
Here, you can see that in output there is no identifier column. We have two non-identifier columns. Each column of the original DataFrame
is now a row in the output DataFrame
.
Now we will pass the optional parameters and check the results.
Example Codes: pandas.melt()
With Single Column as id_vars
import pandas as pd
dataframe = pd.DataFrame(
{
"Attendance": {0: 60, 1: 100, 2: 80, 3: 78, 4: 95},
"Name": {0: "Olivia", 1: "John", 2: "Laura", 3: "Ben", 4: "Kevin"},
"Obtained Marks": {0: "90%", 1: "75%", 2: "82%", 3: "64%", 4: "45%"},
}
)
dataframe1 = pd.melt(dataframe, id_vars=["Name"])
print(dataframe1)
Output:
Name variable value
0 Olivia Attendance 60
1 John Attendance 100
2 Laura Attendance 80
3 Ben Attendance 78
4 Kevin Attendance 95
5 Olivia Obtained Marks 90%
6 John Obtained Marks 75%
7 Laura Obtained Marks 82%
8 Ben Obtained Marks 64%
9 Kevin Obtained Marks 45%
The identifier column is specified as Name
, and the variable
and value
columns are next to it with the values extracted from the original dataframe.
We could also assign names of var_name
and value_name
to replace the default variable
and values
.
import pandas as pd
dataframe = pd.DataFrame(
{
"Attendance": {0: 60, 1: 100, 2: 80, 3: 78, 4: 95},
"Name": {0: "Olivia", 1: "John", 2: "Laura", 3: "Ben", 4: "Kevin"},
"Obtained Marks": {0: "90%", 1: "75%", 2: "82%", 3: "64%", 4: "45%"},
}
)
dataframe1 = pd.melt(
dataframe, id_vars=["Name"], var_name="Performance", value_name="Success"
)
print(dataframe1)
Output:
Name Performance Success
0 Olivia Attendance 60
1 John Attendance 100
2 Laura Attendance 80
3 Ben Attendance 78
4 Kevin Attendance 95
5 Olivia Obtained Marks 90%
6 John Obtained Marks 75%
7 Laura Obtained Marks 82%
8 Ben Obtained Marks 64%
9 Kevin Obtained Marks 45%
Example Codes: pandas.melt()
With Skipping Columns
If we want to check the attendance only, we need to specify the value_vars
.
import pandas as pd
dataframe = pd.DataFrame(
{
"Attendance": {0: 60, 1: 100, 2: 80, 3: 78, 4: 95},
"Name": {0: "Olivia", 1: "John", 2: "Laura", 3: "Ben", 4: "Kevin"},
"Obtained Marks": {0: "90%", 1: "75%", 2: "82%", 3: "64%", 4: "45%"},
}
)
dataframe1 = pd.melt(
dataframe,
id_vars=["Name"],
value_vars="Attendance",
var_name="Performance",
value_name="Success",
)
print(dataframe1)
Output:
Name Performance Success
0 Olivia Attendance 60
1 John Attendance 100
2 Laura Attendance 80
3 Ben Attendance 78
4 Kevin Attendance 95
It only shows the information of the Attendance
column in the original dataframe.
Example Codes: pandas.melt()
With Multiple Columns
We add an extra column ID
to the demo dataframe.
import pandas as pd
dataframe = pd.DataFrame(
{
"Attendance": {0: 60, 1: 100, 2: 80, 3: 78, 4: 95},
"ID": {0: 1, 1: 2, 2: 3, 3: 4, 4: 5},
"Name": {0: "Olivia", 1: "John", 2: "Laura", 3: "Ben", 4: "Kevin"},
"Obtained Marks": {0: "90%", 1: "75%", 2: "82%", 3: "64%", 4: "45%"},
}
)
dataframe1 = pd.melt(dataframe, id_vars=["ID", "Name"])
print(dataframe1)
Output:
ID Name variable value
0 1 Olivia Attendance 60
1 2 John Attendance 100
2 3 Laura Attendance 80
3 4 Ben Attendance 78
4 5 Kevin Attendance 95
5 1 Olivia Obtained Marks 90%
6 2 John Obtained Marks 75%
7 3 Laura Obtained Marks 82%
8 4 Ben Obtained Marks 64%
9 5 Kevin Obtained Marks 45%
Both ID
and Name
columns are assigned as the identifier columns.